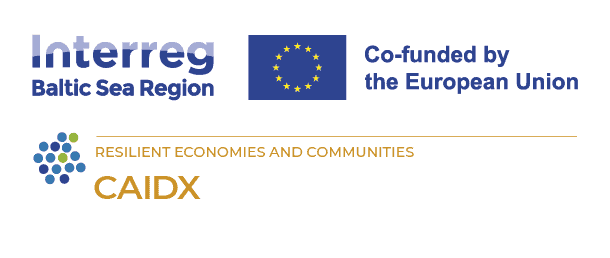
The Importance of Change Management in Introducing AI-Based Diagnostics in Hospitals
05 February 2025
Objective
The objective of this paper is to give an overview of change management concepts for the clinical implementation of AI-based diagnostics and in particular the introduction of the CAIDX implementation and change management guide (CAIDX ICM guide).
Introduction
As artificial intelligence (AI) transforms the landscape of healthcare, AI-based diagnostic tools are poised to revolutionize the way hospitals operate. These innovations promise increased accuracy, efficiency, and personalized care. However, their successful implementation hinges on more than just technological readiness; effective change management is crucial to integrate AI diagnostics into hospital workflows seamlessly.
Introducing AI into hospitals often meets resistance from healthcare professionals who fear job displacement or distrust machine-generated decisions. Change management helps address these concerns by engaging stakeholders early, fostering transparency, and demonstrating how AI complements rather than replaces human expertise. Through structured communication and education, clinicians can see AI as an ally that enhances their diagnostic capabilities.
A Short History of Change Management
Change management, as a formal discipline, emerged in the mid-20th century to address the growing complexities of organizational transformations. Its roots can be traced through various phases of development. In 1947 Lewin introduced one of the first structured models for understanding organizational change. His Unfreeze-Change-Refreeze model (2) emphasized the need to disrupt existing norms, implement new behaviours, and solidify them into a stable state. This era focused on behavioural psychology and group dynamics, laying the groundwork for understanding how people react to change. As organizations grew in size and complexity, scholars like John Kotter and Warren Bennis expanded the focus of change management to include leadership and organizational behaviour. Systems Theory also emerged during this time, emphasizing the interconnectedness of organizational elements and the need for a holistic approach to change. In 1995, John Kotter’s 8-Step Process for Leading Change (3) became a cornerstone of change management, emphasizing the role of leadership, vision, and employee engagement. The ADKAR Model (Awareness, Desire, Knowledge, Ability, Reinforcement) by Prosci (4) emerged as a practical tool for managing individual and organizational change.
Change management in the clinical context
Change management in hospitals is increasingly recognized as a necessity and is more common than it was a decade ago. However, its success and prevalence still depend on the institution’s size, leadership, resources, and culture. In larger systems or progressive hospitals, it’s fairly routine; in smaller or resource-strapped settings, it is still rare. An overview of change management models which are applied in a health care context can be found in a systematic review of Harrison et. al. (5). The review identified several key change management methodologies used in healthcare contexts. The most commonly used methodologies included Kotter’s model, reported in 19 studies, and Lewin’s model, reported in 11 studies. In total, the review identified 12 different change management methodologies used in healthcare settings in 38 studies. These methods were used at different levels, including local ward or unit level, institutional level and system or multi-system level. Hospodková et al. evaluate systemic change management in five European hospitals (6) while the article from Phillips and Klein (7) identifies 15 common strategies found in 16 different change management models and frameworks. Their findings suggest that strategies related to communication, stakeholder involvement, encouragement, organizational culture, vision, and mission should be used when implementing organizational change.
Change management and artificial intelligence
The rise of artificial intelligence, big data, and digital transformation has redefined the scope of change management. Modern frameworks emphasize adaptability, employee-centric approaches, and ongoing innovation. Organizational culture, emotional intelligence, and stakeholder engagement are increasingly viewed as critical to successful change initiatives. Researchers and Healthcare professionals around the globe are struggling with the implementation of novel AI-based tools in the clinical setting. While many different implementation and change management frameworks for the introduction of new technologies in healthcare have been developed, the introduction of AI seem to create unique challenges. In the literature several frameworks for implementation and change management for introducing AI have been published. Here is a brief overview of some of them.
In the paper “Creating a Practical Transformational Change Management Model for Novel Artificial Intelligence-Enabled Technology Implementation in the Operating Room.”, Smith et. al. (8) present an innovative approach to change management (CM) specifically tailored for implementing advanced artificial intelligence technologies in the operating room setting. The authors meticulously define key phases of technology implementation, analyze existing CM models, and establish a new model based on their real-world experiences over the course of a year. Key strategies emphasized in this comprehensive study include team assembly, stakeholder engagement, and the importance of agility and communication in navigating institutional challenges.
The study conducted by Sriharan et al. provides a comprehensive examination of the leadership required for AI transformation in healthcare organizations (9). The findings highlight the multidimensional nature of leadership necessary to effectively navigate the complexities of AI integration and to leverage its transformative potential. Key elements identified include the necessity for leaders to possess an understanding of data quality, risk assessment, and to treat AI as a new team member. Furthermore, leaders should communicate clear business objectives, prepare their teams for change, manage data resources, and foster collaboration across different functional domains—technological, strategic, operational, and organizational.
The CAIDX Implementation and Change Management Guide (CAIDX ICM Guide)
The primary goal of the INTERREG Baltic Sea Region Project CAIDX (Clinical Artificial Intelligence-based Diagnostics) (1) is to establish cooperation between artificial intelligence (AI) providers and healthcare institutions to help healthcare professionals integrate AI, thereby improving diagnostics and treatment.
To achieve this, CAIDX focuses on:
- Developing tools and guidelines to support the development and implementation of AI solutions within healthcare.
- Facilitating contracting, clinical and technical development, capacity building, and collaborations between hospitals, healthcare providers, and the industry ecosystem.
By collecting best practices and creating guidelines for the development, testing, and implementation of AI applications in healthcare, CAIDX supports and accompanies the cultural change necessary to unfold the potential of AI in the healthcare sector.
Change management (CM) is an important, often overlooked corner stone for successful implementation of new technologies in healthcare. In the case of artificial intelligence, CM is of particular importance, due to the ethical, regulatory, legal, and human factors that surround the implementation challenges. Consequently, change management (CM) constitutes a pivotal component within the overarching implementation framework. To this end, the CAIDX project has developed the Implementation and Change Management Guide[1], which is designed to provide change managers with the necessary tools and guidance to optimise their effectiveness in implementing these changes.
The “CAIDX Implementation and Change Management Guide” serves as a comprehensive resource for implementation managers tasked with integrating AI solutions within clinical settings. Aimed at facilitating a smooth transition to AI-enhanced healthcare, this guide outlines essential principles, strategies, and practical steps based on Kotter’s 8-step change management model. It emphasizes the importance of involving end-users from the outset, fostering effective communication, and addressing potential risks throughout the implementation process. By providing a structured approach to planning, executing, and assessing AI integration, the guide aims to empower organizations to navigate the complexities of change management and achieve successful outcomes in their AI initiatives.
Here are the key principles outlined in the CAIDX ICM Guide for successful AI implementation:
- Leadership and Responsible Oversight: Success of implementation an adoption hinges on top management’s alignment and active engagement. Additionally, in is important to appoint a responsible person to oversee the implementation and change management process.
- End-User Involvement and Super-users: Involve end-users from the beginning of the development process to gather insights into their specific needs and ensure the AI technology is accessible in their daily tools. Use Super users or Role Models to lead the implementation process.
- Understanding the Technology: Ensure that end-users understand how the AI technology works conceptually and how it will impact their work processes.
- Early Communication: Start communicating the intended changes very early in the process to prepare all stakeholders.
- Perspective Inventory: Create an inventory of the different involved groups and discuss their varied perspectives on the change process.
- Mutual Respect: Foster a sense of mutual respect where all voices are heard throughout the implementation.
- Resource Allocation: Ensure sufficient resources and time to support a secure and successful implementation.
- On-the-Job Training: Provide on-the-job training to educate and train personnel involved in using the new AI technology.
- Clear Explanation of Technology: Clearly explain what the new AI technology is, how it operates, and its potential influence on daily practices.
- Addressing Concerns: Establish a process for addressing any concerns related to the outputs or results generated by the AI technology
For a more in-depth view and a strategic framework you are invited to download the CAIDX ICM Guide and use it in your own implementation efforts.
Conclusion
While the promise of AI diagnostics in hospitals is undeniable, the journey from introduction to adoption is fraught with challenges. Change management provides the roadmap to navigate these challenges, ensuring that hospitals not only adopt AI technology but do so in a way that empowers clinicians, improves patient care, and aligns with ethical standards. By prioritizing structured change management, hospitals can harness the transformative potential of AI diagnostics and build a future-ready healthcare system.
References:
- CAIDX [Internet]. Interreg Baltic Sea Region. [cited 2025 Jan 28]. Available from: https://interreg-baltic.eu/project/caidx/
- Cummings S, Bridgman T, Brown KG. Unfreezing change as three steps: Rethinking Kurt Lewin’s legacy for change management. Hum Relat. 2016 Jan 1;69(1):33–60.
- Kotter JP. Leading Change: Why Transformation Efforts Fail. Harvard Business Review [Internet]. 1995 May 1 [cited 2025 Jan 28]; Available from: https://hbr.org/1995/05/leading-change-why-transformation-efforts-fail-2
- The Prosci ADKAR® Model | Prosci [Internet]. [cited 2025 Jan 28]. Available from: https://www.prosci.com/methodology/adkar
- Harrison R, Fischer S, Walpola RL, Chauhan A, Babalola T, Mears S, et al. Where Do Models for Change Management, Improvement and Implementation Meet? A Systematic Review of the Applications of Change Management Models in Healthcare. J Healthc Leadersh. 2021 Mar 12;13:85–108.
- Hospodková P, Berežná J, Barták M, Rogalewicz V, Severová L, Svoboda R. Change Management and Digital Innovations in Hospitals of Five European Countries. Healthcare. 2021 Nov 5;9(11):1508.
- Phillips J, Klein JD. Change Management: From Theory to Practice. Techtrends. 2023;67(1):189–97.
- Smith TG, Norasi H, Herbst KM, Kendrick ML, Curry TB, Grantcharov TP, et al. Creating a Practical Transformational Change Management Model for Novel Artificial Intelligence–Enabled Technology Implementation in the Operating Room. Mayo Clin Proc Innov Qual Outcomes. 2022 Oct 27;6(6):584–96.
- Sriharan A, Sekercioglu N, Mitchell C, Senkaiahliyan S, Hertelendy A, Porter T, et al. Leadership for AI Transformation in Health Care Organization: Scoping Review. J Med Internet Res. 2024 Aug 14;26:e54556.