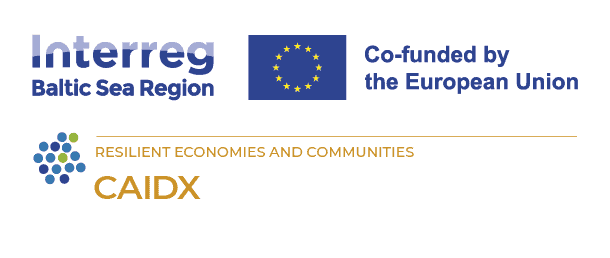
AI in healthcare: The implementation challenge
09 February 2024
The final realisation and implementation of a solution is often not given the same attention as the development. In this short article we argue that a successful implementation is the key step in turning an invention into an innovation. Without successful implementation the investment in research and development is lost.
Publishing a paper in a scientific journal is hard work. Depending on the topic, the review process, the journal and a couple of other factors it may even take up to a year or more. Implementing a new solution in the health care system is even harder.
“In fact, researchers have estimated a 17-year gap from the time that a clinical innovation has proven effective to when it is provided routinely to patients, with only half of evidence-based practices being implemented into care at all”, Kirchner et all. write.
How many companies already have an AI-based product on the market? The database AI for Radiology lists 106 companies with 218 products. How many of these products do you find in a hospital near you? This is hard to tell, but most probably not many.
It seems that getting AI into day-to-day healthcare delivery is very hard. This is not a surprise. Unclear legal framework conditions, a difficult regulatory landscape, lack of knowledge on AI on the end-user side, lack of explainability, lack of interoperability, lack of standards, make the integration of AI solutions into the clinical workflow a nightmare.
Due to the expensive and long certification process, many companies try to avoid developing a product that falls under the Medical Device Regulation (MDR). But even if they have a certified product, it is a steep path to get the product adopted by health care practitioners.
How can we shorten this time-consuming and resource-intensive path? Well, we can ask ChatGPT. May be AI knows how to best implement itself into the clinical workflow. Hey, ChatGPT: “What are the steps to implement an AI algorithm in a clinical workflow? ”
Answer by ChatGPT: “Hello! Implementing an AI algorithm in a clinical workflow involves several steps. First, it’s important to identify the specific problem or task that the AI algorithm will address, such as diagnosing a certain condition or predicting patient outcomes. Then, you’ll need to gather and prepare the necessary data, which may include medical records, lab results, and imaging scans.
Next, you’ll need to choose an appropriate AI algorithm that suits the problem at hand. This could involve using machine learning techniques like deep learning or decision trees. Once you have selected the algorithm, you’ll need to train it using the prepared data. This typically involves feeding the algorithm with labelled examples to help it learn patterns and make accurate predictions.
After training, you’ll need to evaluate the performance of the AI algorithm using validation data. This step helps ensure that the algorithm is reliable and provides accurate results. If the performance is satisfactory, you can move on to integrating the algorithm into the clinical workflow. This may involve working closely with healthcare professionals to ensure seamless integration and addressing any concerns or challenges that arise.
Lastly, continuous monitoring and refinement of the AI algorithm are crucial to ensure its effectiveness and adaptability to changing clinical needs. Regular updates and improvements based on feedback from healthcare professionals and patients can help optimize its performance over time.
I hope this information helps! Let me know if you have any further questions.”
No, this information does not help you much. It is a bunch of common knowledge and obvious steps.
There is definitely not a one-size-fits-all solution or a recipe how to implement AI into clinical practice, but for sure we can come up with a solution that is much more helpful. In a previous project we have developed a framework for Biomarker Comercialization, a process from idea to market that entails 7 phases and more than 130 tasks for a multidisciplinary team organized around a clinical a technical and a commercial perspective.
Yes, this looks complex. But it is a complex process. In Biomarker development it may take up to 10 years from idea to market and the financial investments required for developing and commercializing a new biomarker-based diagnostic technology are substantial, often exceeding $100 million.
The co-development of an AI-based diagnostic method from idea and clinical need definition to a marketable product may be of similar complexity. In contrast to biomarker development, AI-development is a new field where evidence-based practice is rare.
However, the only way of dealing with the complexity is organizing the process in manageable tasks. Helpful is also adopting the perspective of the involved stakeholder groups, namely the clinical, the technical and the business perspective and translating their needs for the other groups to create a common understanding.
If you have experience in the implementation of AI in a clinical setting or you are facing challenges, we would love to hear from you. The CAIDX project welcomes experience from the field, no matter whether it is from a successful use case or a challenge you are struggling with.
With the help from experts and experience from the field CAIDX intends to contribute useful tools that support the implementation of AI in a clinical setting and thus helping to turn a great invention in a successful innovation.
Text by: Thomas Karopka, Senior Project Manager at BioCon Valley GmbH